.png)
What’s ‘the Edge’?
Working 'at the edge' refers to the practice of processing, analyzing and taking action from data on local devices, such as smartphones, cameras, or other Internet of Things (IoT) devices, rather than relying solely on centralized servers or cloud-based systems.
Great news, artificial intelligence (AI) is no longer just confined to the cloud. It is increasingly being deployed to the edge, closer to the sources of data and where decisions need to be made in real-time.
There are a number of reasons for this shift to the edge. First, edge devices are becoming more powerful and capable. This makes it possible to run AI models on edge devices without sacrificing performance. Second, edge devices are often located closer to the sources of data. This reduces latency and improves bandwidth efficiency. Third, edge devices can operate even if the connection to the cloud is lost. This improves reliability and resilience.
.png)
Our founders spent the past decade working with enterprises focusing heavily on commercial drone integration, we saw the potential that was starting to be realized on creating intelligent edge devices. If we know what information we are looking for AND what to do if we find it, why don’t we put that contextual intelligence right on the device collecting data to ensure better, faster decision making?
This has historically been a process that required a team of developers to create hard coded apps for each use case, so a device has both deep tech capabilities and the business context. This is what prompted the creation of Cloneable, a way to combine deep tech and logic together and immediately bring that intelligence to the device.
What does AI moving to the edge mean?
Traditionally, AI models have been trained and deployed on centralized cloud platforms. This approach, while effective, presents limitations in terms of latency, bandwidth constraints, and security concerns. Edge AI addresses these challenges by bringing AI processing and decision-making closer to the data source, at the edge of the network.
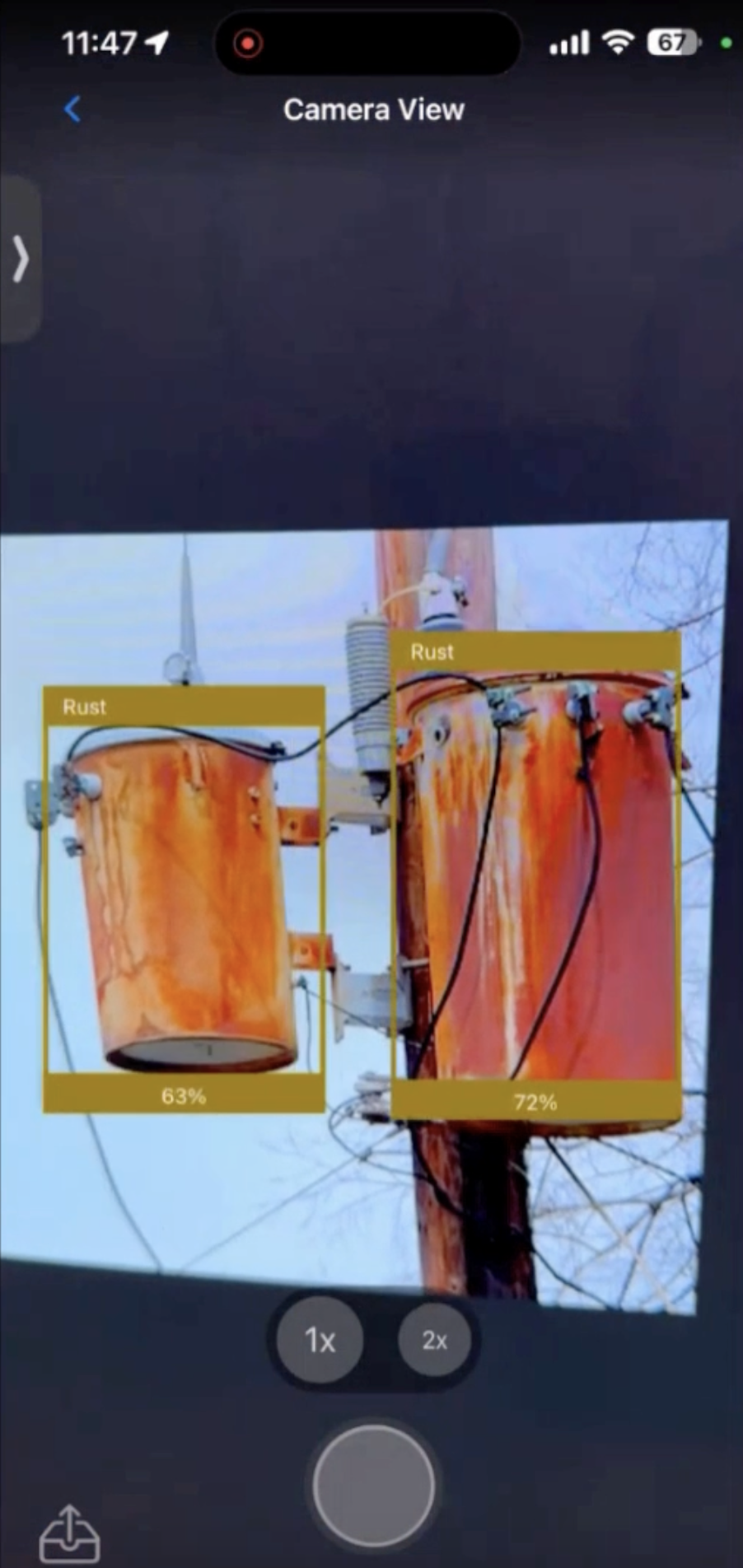
Why is AI moving to the edge?
Several factors are driving the movement of AI to the edge:
- Reduced latency: Edge AI enables real-time decision-making and response, eliminating the need for data to travel to and from central servers. This is crucial for applications that require immediate action, such as autonomous vehicles and industrial automation.
- Bandwidth optimization: Edge AI reduces the amount of data that needs to be transmitted to the cloud, minimizing network congestion and bandwidth costs. This is particularly beneficial for applications that generate large volumes of data, such as video surveillance and IoT devices.
- Improved security: Edge AI enhances data privacy and security by keeping sensitive data localized. This reduces the risk of data breaches and unauthorized access, particularly in industries with strict compliance requirements.
- Cost: The cost of cloud storage to move and process huge amounts of data on a daily basis is a major expense. Using drones as an example again, each asset requires multiple images and often video to properly inspect, and often this must be repeated over thousands of assets daily and sent to the HQ for analysis.
Key Benefits of Edge AI
Edge AI offers a multitude of benefits beyond reduced latency, bandwidth optimization, and enhanced security:
- Autonomy and resilience: Edge AI systems can operate independently, even in the event of network disruptions or cloud outages. This ensures continuous operation and minimizes downtime.
- Scalability and flexibility: Edge AI deployments can be easily scaled to accommodate growing data volumes and evolving application requirements. This allows businesses to adapt quickly to changing needs.
- Personalized experiences: Edge AI enables real-time analysis of user behavior and preferences, allowing for personalized recommendations, targeted advertising, and adaptive user interfaces.
Empowering Businesses with Edge AI Tools
AI deployment has historically been accessible to developers and data scientists with a particular set of knowledge and skills, particularly in the areas of ML Ops, model training and coding to bring their model to the real world in custom apps. However, the emergence of powerful platforms like Scale AI, Landing AI, Roboflow, LabelBox, and Ultralytics has revolutionized AI workflows, unlocking unprecedented access and value.
.png)
In fact, the transition to edge AI is fueled by a growing ecosystem of tools and technologies that simplify development, deployment, and management of edge AI solutions. These tools include:
- Edge computing platforms: Provide the hardware and software infrastructure to run AI models at the edge.
- AI development tools: Enable the creation and optimization of AI models for edge deployment.
- Edge AI management tools: Help to monitor, troubleshoot, and update edge AI devic deployments.
From very specific business needs like ‘rust identification’ or ‘car damage’ to analyzing human experiences like pose estimation, the democratization of AI-based tools is a rapidly advancing movement that aims to make deep tech more accessible to nontechnical users. This is being achieved through the development of user-friendly interfaces, drag-and-drop functionality, and pre-trained models that can be easily adapted to specific needs.
By democratizing the tedious processes of labeling, training, and monitoring computer vision models, businesses can generate high-performing, accurate models tailored to specific application needs, accelerating the development cycle and unlocking new possibilities.
However, how these models are deployed and applied in the real world presents a new set of challenges. While these platforms excel at expediting the transition from development to deployment, applying these models to align with specific business rules and seamlessly integrating them into existing business systems often require significant coding and development effort.
The ability to personalize and run AI at the edge is where Cloneable is already transforming various industries with its ability to deliver real-time insights and actions.
Cloneable streamlines the application of models but also seamlessly connects them to an array of business logic without the need for code. This innovative tool eliminates the friction associated with model application, enabling developers to create agile and adaptive systems that can readily adapt to diverse business goals and decision logics without delving into complex coding intricacies.
Real-World Applications of Edge AI
In the following video, our team demonstrates three real-world applications of edge AI:
- Scanning a job site to ensure everyone is wearing the mandated protective gear
- Identifying rust and the amount of rust on a utility transformer
- Counting, analyzing and pricing an agricultural commodity to determine the amount to pay a vendor.
The movement of AI to the edge marks a significant step forward in the evolution of AI technology. By unleashing the power of distributed intelligence, edge AI is paving the way for a future of real-time insights, autonomous decision-making, and enhanced security. Organizations that embrace edge AI will be well-positioned to innovate, adapt, and thrive in the rapidly changing technological landscape.